How good are the titrations?
In order to get an idea of the agreement between the results of fifteen students, each having done three replicate measurements, one could count how many titration results are not further away than 0.005 M from the mean value of 0.1005 M. You can verify this by clicking on the "Submit" box below. Next, find how many results differ less than 0.0017 M, the standard deviation, from the true value. How many are closer to the mean value than 2 times and 3 times the standard deviation?
The reverse procedure is also possible. Using the next "Submit" button, you can calculate what interval around the mean value contains 95% of all titration results.
From the past to the future...
The principal idea in statistics is the notion that more or less the same results would be expected if the same group of students would perform the same titrations again. Although the individual results would be different, the distribution of the results would be similar. The distribution of the measurements is often approximated by a normal distribution. This is completely defined by only two values: the mean and the standard deviation of the distribution. The spread around the mean value, as measured by the standard deviation, is directly related to the width of a prediction interval. Now, what is a prediction interval anyway?
Prediction intervals
A prediction interval of 95 percent simply means that we expect 95% of all future measurements to fall within this interval. This also means that 5% of all measurements are expected to fall outside! Likewise, prediction intervals of 90% and 99% are often used. The exact calculation of a prediction interval requires a bit of background which is beyond this course; however, approximate values for prediction intervals can easily be explained. We already hinted that the width of a prediction interval is related to the standard deviation of the data. Now, as a rule of thumb, a prediction interval of 95% is obtained by taking the mean plus or minus twice the standard deviation. A prediction interval of 99% (approximately) is given by the mean plus or minus three times the standard deviation:
Question: why are 99% prediction intervals wider than 95% prediction intervals?
We now see that prediction intervals routinely are constructed from previous data. This implies that the intervals are only valid if we expect the future data to behave in the same way!
The limit of detection
A direct application of prediction intervals is the determination of the limit of detection (LOD) of quantitative analytical methods. A definition of the LOD is: the LOD is the smallest signal value that is significantly (e.g. with 99% confidence) different from the signal of a true blank. To assess the LOD, a sufficient number of true blank values should be measured (preferably more than 20). The LOD is then equal to the mean signal of these measurements plus three times the standard deviation.
Using this procedure (because the LOD is the upper bound of a 99% prediction interval), you are 99% sure that a sample yielding a larger signal value than the LOD is not a blank, so the signal is actually due to analyte.
Confidence intervals
A 95-percent prediction interval implies that there is a 95 percent chance that another titration experiment would find a value in that range (provided it is executed in exactly the same way as all the other volume determinations, and by the same people).
However, each student performed 10 volume determinations, and took the mean value of these as the final result. Obviously, the histogram of all these mean values shows considerably less variation than the individual volume determinations (remember, errors cancel out!). This means that the standard deviation of a mean value is smaller than the standard deviation for individual values.
The histograms of the individual measurements and the mean values are depicted below.
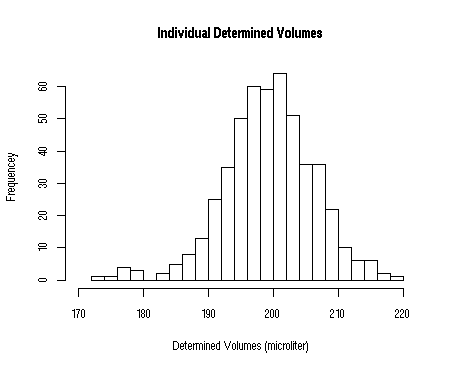
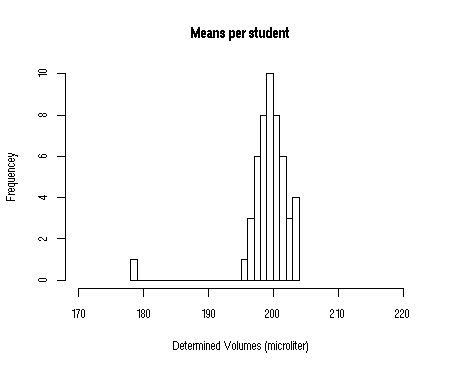
where is the number of measurements used to calculate the mean.
, the Greek lowercase letter sigma, is often used as the symbol for the standard deviation and
, the Greek lowercase letter mu, as the symbol for the mean (but the latter one does not occur in the equation).
Confidence intervals are calculated in exactly the same way as prediction intervals for individual measurements, only the standard deviation for the mean is used instead of the standard deviation of the individual measurements. This formula also explains why the mean is more precise when we use more data: its prediction interval becomes narrower. Again, note that this does not mean that the standard deviation of the individual measurements gets smaller!
Now, continue with the questions on this subject.